Exploring the Mechanics of Audio-to-Text Technology
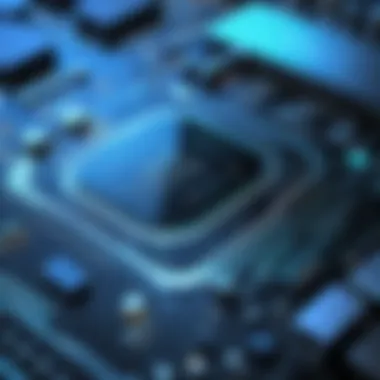
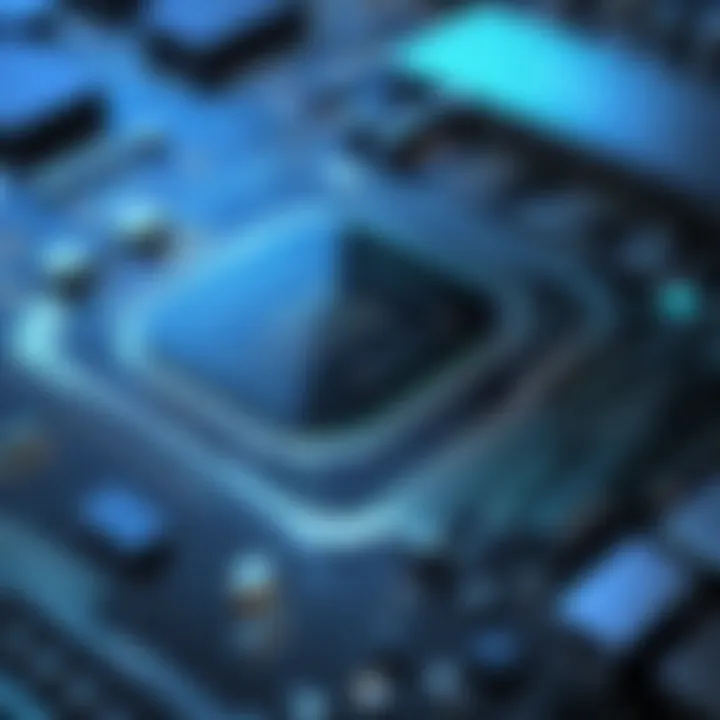
Intro
Audio-to-text transcription programs have emerged as essential tools across various fields. These systems convert spoken language into written text. This transformation opens diverse avenues in sectors like media, legal, and academic. The technology behind these tools relies on complex algorithms that enable speech recognition and processing.
As the demand for efficient transcription services rises, understanding the mechanics of these programs becomes crucial. For IT professionals and tech enthusiasts, knowing how these systems function enhances their ability to implement and utilize them effectively. This article aims to dissect the intricacies of audio-to-text transcription technology. It will explore topics such as performance metrics, usability, and future trends shaping the industry.
Performance Metrics
Effective audio-to-text transcription hinges on performance metrics. Tracking these metrics provides insights into how well the systems work. They include accuracy, speed, and reliability, which are critical for evaluating any transcription tool's effectiveness.
Benchmarking results
Accuracy is a key performance metric for these programs. Transcription systems typically achieve varying levels of accuracy based on factors like speech clarity, background noise, and language variations. Studies have shown that leading transcription software can attain accuracy rates exceeding 90%. Advanced models employing machine learning methodologies often outperform their traditional counterparts in handling diverse accents and dialects.
In terms of benchmarking, performance comparisons among several programs are integral. Evaluating platforms like Google Speech-to-Text, IBM Watson, and Microsoft Azure can give valuable insights. Each of these services offers a unique approach, but performance can differ significantly based on the context of use.
Speed and responsiveness
Speed is another critical aspect of transcription programs. Real-time transcription capabilities are particularly valuable in live settings, such as conferences or courtrooms. Systems that lag can disrupt communication and cause frustrations. An ideal transcription program should respond promptly, ensuring smooth interaction.
Utilizing metrics such as words per minute (WPM) helps gauge responsiveness. Those clocking faster WPM rates generally indicate better performance. Monitoring the response time can inform decisions on the most suitable software for specific tasks.
Usability and User Experience
Beyond performance, a transcription program's usability directly influences user satisfaction. If a tool is difficult to install or navigate, even the most advanced technology may underperform.
Ease of installation and setup
Effective audio-to-text transcription tools must offer straightforward installation processes. Complex setups often deter users and lead to suboptimal use of the software. Clear documentation is essential, providing guidance on installation and configuration.
A good installation experience sets a positive tone for user engagement. When individuals can set up software quickly, they are more likely to explore additional features and capabilities.
Interface design and navigation
After installation, the interface design becomes a focal point for user experience. An intuitive layout enhances navigation. Users should be able to locate features effortlessly without facing steep learning curves.
Modern transcription software often employs user-centric design principles. For instance, familiar icons and well-organized menus contribute to a seamless experience. Ensuring that user experience remains at the forefront is vital for retention and satisfaction.
"The success of audio-to-text transcription programs lies not just in their technology, but in their usability."
Future sections will delve into more technical aspects and trends shaping the landscape of transcription software.
Prelims to Audio-to-Text Transcription
Audio-to-text transcription has emerged as a critical component in various fields today. The importance of this technology lies in its ability to convert spoken language into written text accurately and efficiently. This capability is being utilized in media, legal, academic, and numerous other sectors. Therefore, understanding the mechanics behind these transcription systems is essential for IT professionals and tech enthusiasts.
One of the main benefits of audio-to-text transcription is its efficiency. Manual transcription can be labor-intensive and time-consuming. In contrast, modern transcription programs speed up this process significantly, allowing for more time to focus on analysis and decision-making rather than basic data entry. The accuracy or reliability of such systems is also a focal point, as advancements in technology lead to fewer errors in transcription. This is especially important in professional settings, where the stakes can be high.
Moreover, there are several considerations when integrating transcription programs into various workflows. IT professionals must assess the specific needs of their organizations and choose solutions that align with these objectives. Factors like user experience, customization, and integration capabilities with existing systems are crucial for ensuring seamless adoption.
"The future of audio-to-text transcription is closely linked to advancements in artificial intelligence and machine learning, enhancing its scope and accuracy."
The landscape is evolving rapidly, and staying informed about the latest developments in transcription technology is vital. This article will delve deeper into the mechanics, technologies, and challenges associated with audio-to-text transcription, providing a comprehensive guide for professionals aiming to leverage these tools effectively.
Understanding Transcription
Transcription, in essence, is the systematic translation of spoken words into a textual format. This process may seem straightforward, but it encompasses a range of complexities. Different nuances of language, such as intonations, colloquialisms, and accents complicate the transcription process. Programs must be designed to handle these variances to produce accurate results.
There are two main forms of transcription:
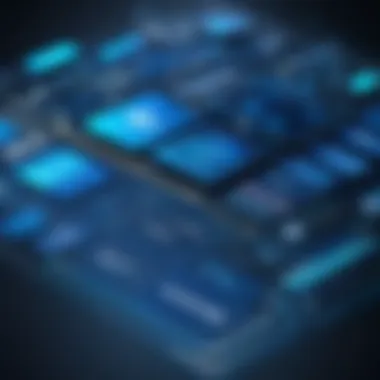
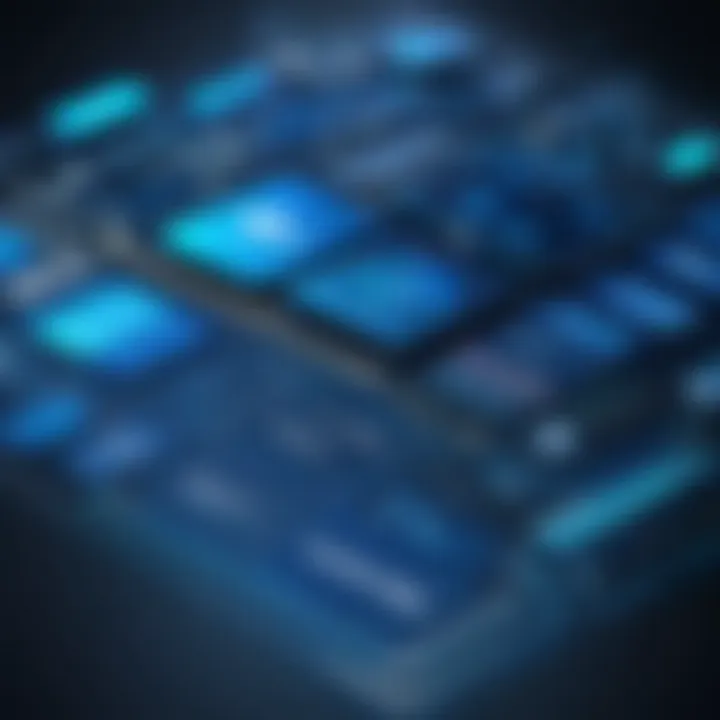
- Manual Transcription: Involving human transcribers who listen to audio recordings and type out what they hear. This method often leads to high accuracy but is slow and can be costly.
- Automated Transcription: Utilizes sophisticated algorithms and machine learning to transcribe audio. This method is much quicker but can struggle with accuracy in challenging audio conditions.
Both methods have their advantages and drawbacks. Understanding these can help organizations choose the right approach for their needs. Accurate transcription not only enhances accessibility but also ensures compliance with legal and regulatory standards in many industries.
As the demand for real-time and accurate audio-to-text services grows, the landscape of transcription technology continues to evolve. The following sections of this article will explore the technology that supports transcription programs, offering deeper insights into how they function and their practical applications.
Technology Behind Transcription Programs
The technological framework of audio-to-text transcription programs is complex yet fascinating. It forms the backbone of how these systems convert spoken language into written text. Understanding this technology is crucial not only for technical professionals but also for industries that rely heavily on accurate transcription services. Factors such as accuracy, speed, and adaptability play significant roles in the feasibility and functionality of these programs.
Many transcription systems integrate various speech recognition algorithms and machine learning techniques to boost performance. Such combinations improve the ability to interpret diverse voices, accents, and environments. As a result, companies can enhance productivity. Moreover, solid technical foundations allow for ongoing improvements and updates to meet evolving user needs.
These transcription programs support real-time usage in applications like customer service and note-taking. The nuances of handling different languages, jargon, and slang further highlight their relevance. Thus, the technology behind these systems holds vast implications for efficiency and quality in communication.
Speech Recognition Algorithms
Speech recognition algorithms serve as the core components that drive transcription software. They analyze audio input and facilitate its transformation into text. Different algorithms yield varying success rates depending on conditions such as the sound environment or the speaker’s accent. This section will explore three pivotal methodologies: Hidden Markov Models, Deep Learning Techniques, and Natural Language Processing.
Hidden Markov Models
Hidden Markov Models (HMM) are statistical models that characterize the probabilities of sequences of observable events. An important aspect of HMM is its capacity to handle time-dependent variables effectively, which benefits audio processing. They are often favored in transcription systems due to their adaptability in recognizing phonemes and generating viable outputs from incomplete information.
The key characteristic of HMM lies in its ability to learn patterns from historical data. This makes it a popular choice for many businesses that prioritize cost-effective solutions. A unique feature of HMM is its capability of accommodating different states of user input. However, one disadvantage could be its requirement of significant initial data for training the models, which may pose challenges for startups or smaller firms.
Deep Learning Techniques
Deep learning techniques utilize neural networks to analyze audio signals, achieving exceptional accuracy in transcription tasks. The ability for these models to improve from vast amounts of data is notable. This learning capacity enhances the user experience, particularly in scenarios with varied accents or speech patterns. Deep learning models are beneficial for scaling applications across diverse industries.
What sets deep learning apart is its sophisticated architecture, mimicking the human brain's functioning. This adaptability leads to higher precision rates during transcription. Nevertheless, deep learning requires substantial computational resources and can be costly to implement effectively.
Natural Language Processing
Natural Language Processing (NLP) involves the interaction between computers and humans through natural language. This aspect is vital for the refined understanding of context and semantics in transcription outputs. NLP is essential for capturing nuances, sentiment, and intent in speech, contributing significantly to the overall goal of accurate transcription.
A key characteristic of NLP is its ability to analyze language patterns and meanings, making it a necessary component of effective transcription programs. It enables systems to offer context-aware improvements and enhance the quality of results. However, challenges exist in understanding cultural references or idiomatic expressions, which can lead to misinterpretations in some cases.
Machine Learning in Transcription
Machine learning techniques are applied progressively within transcription programs. Such algorithms allow systems to become increasingly accurate over time by learning from user interactions and feedback. With the constant evolution of language, machine learning enables programs to adapt quickly to trends and changes in speech use.
The dynamic nature of machine learning provides opportunities for fine-tuning and continuous improvement. Integration of this technology enhances user satisfaction and reduces the margin for error. It also permits customization according to specific industry needs, making it an integral part of ongoing developments in audio-to-text transcription.
Key Features of Transcription Software
In considering audio-to-text transcription software, several key features emerge as critical to their efficacy and usability. This section will explore these features in detail, emphasizing their importance for IT professionals and tech enthusiasts alike.
Accuracy and Reliability Metrics
Accuracy stands as the paramount criterion when evaluating transcription software. High accuracy not only ensures that the text generated reflects the spoken content faithfully but also saves time in post-editing. Transcription programs commonly rely on various metrics to gauge their performance, including word error rate (WER) and sentence error rate (SER).
- Word Error Rate (WER): This measures how many words are incorrectly transcribed compared to the reference transcript. A lower WER indicates higher accuracy.
- Sentence Error Rate (SER): This looks at entire sentence accuracy, providing a broader view of overall performance.
Reliability plays an equally important role. Users must trust that the software consistently delivers high-quality results across various settings. Factors like updates to algorithms and user feedback significantly impact reliability.
User Interface Design Considerations
A user-friendly interface is vital for the adoption of transcription software, particularly for those in fast-paced environments.
- Simplicity and Clarity: The interface should be intuitive, allowing users to navigate features without extensive training.
- Accessibility: Ensure that options are flexible for diverse user needs, including those with disabilities. Features like keyboard shortcuts can greatly enhance usability.
- Feedback Mechanisms: Real-time feedback helps users identify errors quickly, thus improving workflow efficiency.
The design of the user interface directly affects user experience and overall satisfaction. Users should feel empowered, not frustrated, while interacting with the software.
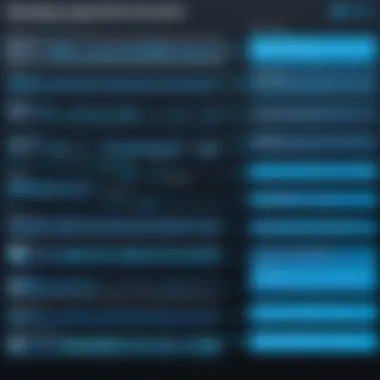
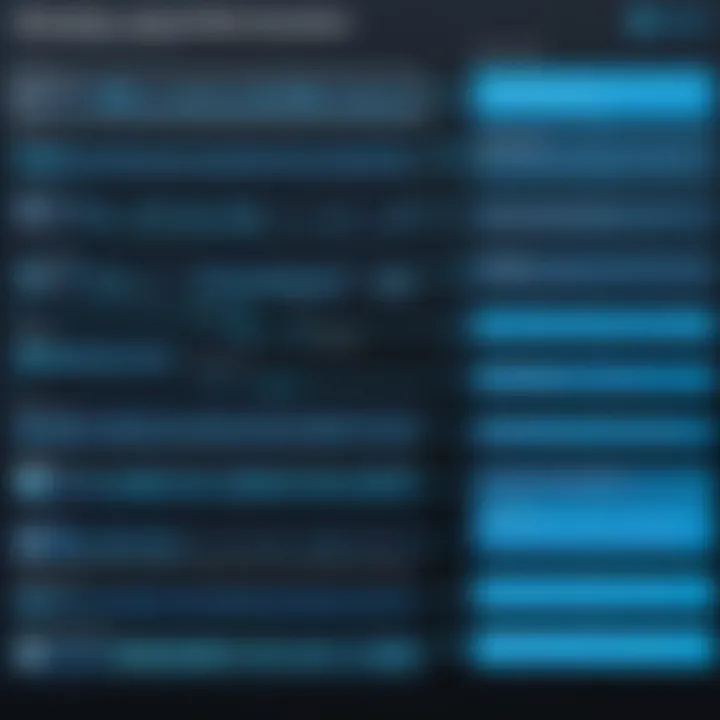
Integration Capabilities
In the current tech landscape, integration with existing systems is a significant consideration for any transcription tool.
Transcription software must efficiently collaborate with other platforms and services. Key points to consider are:
- Cloud Services: Integration with platforms like Google Drive or Dropbox allows seamless access to audio files and storage for the final text documents.
- API Support: Application Programming Interfaces (APIs) enable developers to connect the transcription service with other applications, enhancing functionality.
- Multi-format Support: The ability to handle various audio formats is essential. Compatibility with common file types like MP3, WAV, and others broadens the usability of the software.
Integration capabilities are imperative for ensuring that transcription tools can be embedded within broader workflows, minimizing disruption and maximizing productivity.
Applications of Audio-to-Text Technology
The applications of audio-to-text technology offer profound implications for various sectors. This transformative tool enhances productivity and improves information accessibility. The significance lies in its ability to convert spoken language into written text, which facilitates documentation and review processes. Different fields, including media, legal, and academia, benefit greatly from these systems. An emphasis on accuracy and efficiency in transcription enhances communication, data analysis, and record keeping.
Usage in Media and Journalism
In media and journalism, the demand for rapid content creation and dissemination is high. Audio-to-text transcription technology allows journalists to quickly convert interviews, press conferences, and reports into written articles. Such efficiency is vital in an environment where breaking news must be reported swiftly. Moreover, transcription software aids in creating subtitles and transcripts for broadcasts, thereby enhancing accessibility for audiences with hearing impairments. The speed at which these programs operate can significantly reduce turnaround times and increase output for reporters and content creators.
Implementation in Legal Settings
Legal professionals utilize audio-to-text technology in courtrooms and law offices. Recording deposits and court proceedings is common; however, these audio files require transcription for record-keeping and legal documentation. Using transcription programs streamlines this process, enabling attorneys and paralegals to have immediate access to written records. Not only does this save time, but it also minimizes human error in documentation, which is crucial for maintaining legal integrity. The accuracy of these transcriptions ensures that all spoken words are captured for later reference, thus supporting effective litigation.
Academic and Research Applications
In academia, audio-to-text transcription plays a critical role in research and learning environments. Researchers can record interviews or lectures and quickly have transcripts available for analysis. This efficiency aids in data collection and analysis for various studies. Furthermore, students with disabilities benefit greatly from having lectures transcribed, enhancing their learning experience. The technology supports diverse educational needs by providing materials in formats that are accessible and easier to process. This seamless integration of transcription services into academic practices allows for a more inclusive educational environment.
"The emerging technologies, including audio-to-text transcription, bridge gaps in communication, encouraging broader participation in various fields."
Through these applications, audio-to-text technology not only enhances productivity but also makes communication more inclusive. As the functionality and accuracy of these systems continue to improve, their adoption in different sectors will likely become more widespread. The benefits realized through the effective implementation of these tools emphasize their growing importance in the modern landscape.
Challenges Faced by Transcription Programs
In the advancing field of audio-to-text transcription, various challenges hinder the efficiency and accuracy of these systems. Understanding these challenges is crucial for improving software performance and user satisfaction. Addressing such obstacles creates room for enhancement, allowing transcription programs to be more dependable and applicable across multiple industries.
Variability in Accents and Dialects
One prominent challenge is the variability in accents and dialects. Human speech varies widely based on geographic and cultural factors, which can lead to significant discrepancies in pronunciation and intonation. This variability impairs the effectiveness of transcription programs. When speech recognition algorithms are trained predominantly on specific accents, they may struggle with those from different regions, leading to inaccurate transcriptions.
Organizations must take steps to incorporate diverse datasets for training speech recognition models. This approach enhances the accuracy for a broader range of users. Implementing comprehensive linguistic databases that capture various accents can help bridge this gap. Additionally, ongoing fine-tuning of algorithms allows for further adaptability to different speech patterns. This is a vital consideration for companies that operate internationally or in multicultural environments.
Handling Background Noise
Another challenge is handling background noise. Many audio recordings occur in less-than-ideal environments where external sounds interfere with speech clarity. These can range from street noise to chatter in busy offices. Transcription programs must effectively filter out this noise to deliver clear transcriptions.
Advanced noise-canceling algorithms can assist in isolating voices, but they cannot eliminate noise entirely. Thus, the clarity of audio input remains critical. Users can improve transcription efficiency by ensuring high-quality recordings are used. Understanding the limitations of existing noise-cancellation technologies could lead to better expectations regarding transcription accuracy in noisy environments.
Cost Implications for Implementation
Finally, the cost implications for implementation present a significant challenge. Many organizations must consider the financial investment required to deploy effective transcription systems. This includes costs related to software acquisition, maintenance, and possibly hardware upgrades for optimal performance.
Organizations must analyze the Return on Investment (ROI) when implementing transcription software. In many cases, investing in higher-quality solutions can lead to long-term savings by increasing productivity and minimizing transcription errors. It is also important to factor in training and support costs, as staff must be educated on how to use these programs effectively.
"Understanding and overcoming obstacles in transcription technology is essential for future improvements in its applications across diverse fields."
Evaluating the Performance of Transcription Software
Evaluating the performance of transcription software is crucial in determining its effectiveness in real-world applications. For IT professionals, understanding how these programs perform can guide decisions about technology investments and project implementations. With the rapid developments in audio-to-text technology, choosing the right software is critical. Wrong choices can lead to wasted resources and unsatisfactory outcomes. Evaluating performance involves considering multiple factors.
One major element to assess is accuracy. Accuracy refers to how well the transcription software converts spoken language into text. High accuracy is essential for effective communication, especially in critical sectors like healthcare or legal. Another important consideration is speed. The turnaround time of a transcription service can affect workflow efficiency.
Additionally, evaluating user interface and experience can greatly impact usability. A streamlines UI allows users to navigate the software with ease. These factors collectively enhance the overall performance evaluation process.
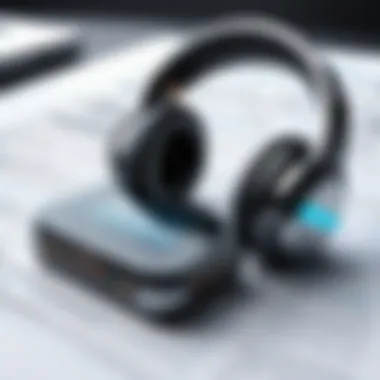
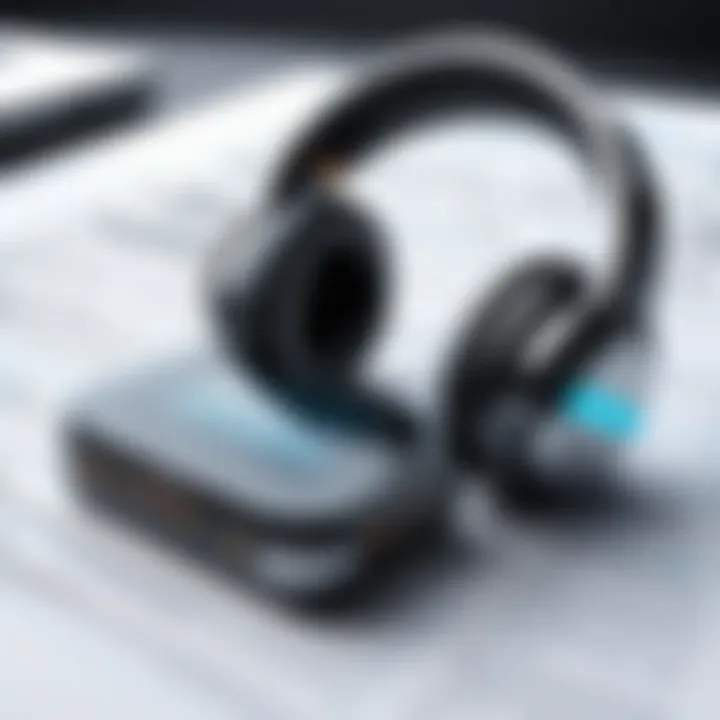
Metrics for Assessment
To effectively measure performance, specific metrics must be applied. Some of the common metrics include:
- Word Error Rate (WER): This measures the percentage of words that were incorrectly transcribed. A lower WER indicates better performance.
- Precision and Recall: Precision evaluates how many words were transcribed correctly out of all words recognized. Recall measures how many words from the original audio were accurately captured. Both metrics provide a comprehensive view of transcription quality.
- Processing Time: This tracks the time taken for the audio to be processed into text. Rapid processing without sacrificing quality is desirable.
- User Satisfaction Score: Gathering feedback from users can provide insights into their experience with the software. High satisfaction ratings indicate that the software meets user needs.
Using these metrics, organizations can establish benchmarks that should be met. Continuous monitoring can help in making necessary adjustments for performance improvements.
User Feedback and Case Studies
User feedback serves as a valuable resource when evaluating transcription software. Analyzing experiences from various users can unveil potential issues that metrics may not capture. Individual use cases often reveal specific strengths and weaknesses in the software. For instance, a media organization may highlight the impact of real-time transcription accuracy on their news reporting pipeline.
Case studies can illustrate results from organizations implementing transcription solutions. These narratives provide contextual data that demonstrate software effectiveness. For example, a legal firm might document an increase in efficiency when using transcription software for case documentation. The ability to convert hours of meeting audio into text can save substantial time and resources.
Collecting user feedback is essential to gain real insights into software performance and applicability.
By focusing on user experiences through case studies combined with quantitative metrics, organizations can create a well-rounded evaluation. Understanding both the technical aspects and user implications is critical for successful integration of transcription technology in any operational environment.
Trends Influencing Future of Transcription Technologies
The realm of audio-to-text transcription technologies is constantly evolving. As we look toward the future, it is crucial to explore the trends that shape its development. Understanding these trends plays a significant role in how IT professionals and tech enthusiasts can plan for implementation and use of these technologies in various sectors.
Advancements in AI and
Artificial intelligence (AI) and machine learning (ML) are pivotal in driving the evolution of transcription programs. These technologies enable systems to learn from vast datasets and improve over time. One significant advancement is the development of more sophisticated algorithms that can understand context better. For instance, deep learning techniques harness neural networks that recognize patterns in data more effectively than traditional methods.
This progress allows for higher accuracy rates in transcription. Systems can now adapt to varying speech patterns, accents, and even emotional tones. Such adaptability is essential for industries where precision is paramount, like legal or medical fields. Furthermore, continual improvements in natural language processing enhance the understanding of human speech nuances. AI and ML will undoubtedly continue to innovate the capabilities of transcription software.
Real-Time Transcription Capabilities
A notable trend is the demand for real-time transcription capabilities. As professionals require immediate access to transcribed data, the development of software providing live output is increasingly vital. This is especially relevant in settings like conferences, interviews, and live broadcasts, where timing is critical.
Real-time transcription involves processing audio inputs and converting them into text instantly. This capability enhances communication and collaboration among teams working in digital environments. With advancements in cloud computing and high-speed internet, the feasibility of providing accurate live transcriptions has improved.
"Real-time transcription not only improves productivity but also enables inclusive practices by making information accessible to more individuals."
The integration of these systems into various applications will further streamline workflows across diverse industries. Future transcription technologies will likely aim for seamless interplay between audio inputs and text outputs, making tasks easier for users and boosting overall efficiency.
In summary, the trends in AI, ML, and real-time transcription are shaping the future of these technologies. As systems become more advanced and capable, they hold promise for enhanced productivity and greater accessibility in various settings.
Future of Audio-to-Text Transcription Programs
The future of audio-to-text transcription programs is an essential aspect of understanding the trajectory of transcription technology. As the demand for accurate and efficient transcription solutions grows, it becomes increasingly clear that advancements in artificial intelligence and machine learning will significantly shape this field. IT professionals and technology enthusiasts must pay attention to these developments, as they promise to enhance the capabilities and applications of transcription systems.
Potential Innovations
One of the primary areas of innovation lies in the integration of advanced artificial intelligence algorithms, including deep learning and neural networks. These innovations are likely to lead to improvements in
- Accuracy: Enhanced algorithms can improve the recognition of diverse accents and dialects. This increased accuracy can broaden the usability of transcription services across different markets and regions.
- Contextual Understanding: Natural language processing can better comprehend contextual elements, making transcriptions more meaningful and relevant.
- Real-Time Capabilities: Future systems may provide seamless real-time transcription, which is particularly valuable in live events, classrooms, and meetings.
An emphasis on user experience will also lead to new interface designs that simplify the interaction with transcription software. This could include voice-activated commands and customizable settings tailored to individual user needs.
Moreover, the potential rise of cloud-based solutions will facilitate accessibility and collaboration. With more organizations opting for remote work solutions, transcription tools that offer cloud integration will enable teams to collaborate efficiently, regardless of their geographic locations.
"The next generation of audio-to-text transcription programs will redefine versatility in transcription across industries, guiding easier access to information."
In summary, the future of audio-to-text transcription programs will be characterized by substantial innovations and enhancements. These advancements will unlock new capabilities, enhance user engagement, and broaden the applicability of transcription technology, making it an invaluable tool across various sectors.
End
The conclusion of any comprehensive examination serves as a vital element to encapsulate and reinforce the key points discussed throughout the work. In this context, the conclusion of the article about audio-to-text transcription programs draws attention to significant insights and observations that emerge from the detailed exploration of this technology. For IT professionals and tech enthusiasts, it emphasizes the importance of understanding how transcription software operates and its diverse applications.
Summarizing Key Insights
The discussion in this article highlights critical aspects of audio-to-text transcription technology:
- Technology Underpinnings: Understanding the role of speech recognition algorithms, such as Hidden Markov Models and deep learning techniques, underscores how these systems function. The mathematical and computational foundations of these algorithms determine the overall accuracy and efficiency of transcription.
- Application Diversity: The versatility of transcription programs spans various sectors, including media, legal, and academia. Each application has unique requirements and challenges, prompting developers to tailor their tools accordingly.
- Performance Challenges: Acknowledging challenges such as background noise and variability in accents or dialects is crucial. These factors can affect the accuracy of transcription and must be addressed to enhance the overall performance.
- Future Trends: The future of transcription technology hinges on advancing artificial intelligence and machine learning capabilities. Innovations will shape real-time transcription solutions, making them more efficient and widely adopted.